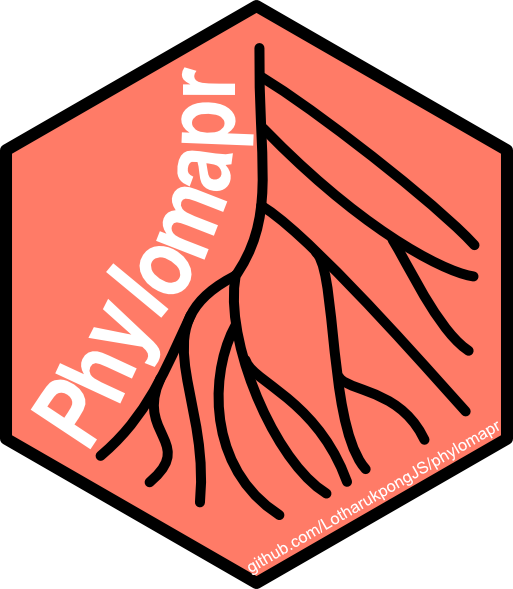
Gene names in different databases
2023-10-23
GeneIDs.Rmd
Motivation
The GeneID labels differ across databases. This is a potential problem when gene age inference has been performed from protein sequences obtained from one database (e.g. UniProtKB) and the RNA-seq quantification has been performed on genes from another database (e.g. ENSEMBL).
Solution 1
In phylomapr
, we try to mitigate this issue using the
helper function convertID()
, which essentially wraps around
the function biomart()
in the biomartr
package. In this article, we give an example of how C. elegans
phylomaps can be converted from the uniprot format to the wormbase
format.
Typically, the gene IDs can be converted to the ENSEMBLE gene IDs,
whose biomartr
attributes are ensembl_gene_id
and ensembl_peptide_id
by default.
# default run of convertID
Caenorhabditis_elegans.PhyloMap.ENSEMBL <-
phylomapr::convertID(
phylomap = phylomapr::Caenorhabditis_elegans.PhyloMap,
mart = "ENSEMBL_MART_ENSEMBL",
dataset = "celegans_gene_ensembl",
filters = "uniprot_gn_id"
)
If you run this, the gene IDs are named as
e.g. WBGene00000001
. However, gene IDs in other RNA-seq
experiments employ other gene names. In the case of C. elegans
this may be wormbase IDs (e.g. 2L52.1a
). How can we convert
the phylomaps to accommodate this?
First, the organismFilters
function in
biomartr
can be used to obtain the attributes. Here, we ask
for the topic worm
because we are interested in wormbase
IDs
c_elegans_BM.filt <- organismFilters(organism = "Caenorhabditis elegans", topic = "worm")
c_elegans_BM.filt
#> # A tibble: 72 × 4
#> name description dataset mart
#> <chr> <chr> <chr> <chr>
#> 1 with_wormbase_cds With WormBase CDS ID(s) celegans_gene_ensembl ENSEMBL_MART_ENSEMBL
#> 2 with_wormbase_gene With WormBase Gene ID(s) celegans_gene_ensembl ENSEMBL_MART_ENSEMBL
#> 3 with_wormbase_gseqname With WormBase Gene Sequence-name ID(s) celegans_gene_ensembl ENSEMBL_MART_ENSEMBL
#> 4 with_wormbase_locus With WormBase Locus ID(s) celegans_gene_ensembl ENSEMBL_MART_ENSEMBL
#> 5 with_wormbase_transcript With WormBase Transcript ID(s) celegans_gene_ensembl ENSEMBL_MART_ENSEMBL
#> 6 with_wormpep_id With Wormpep ID ID(s) celegans_gene_ensembl ENSEMBL_MART_ENSEMBL
#> 7 wormbase_cds WormBase CDS ID(s) [e.g. 2L52.1a] celegans_gene_ensembl ENSEMBL_MART_ENSEMBL
#> 8 wormbase_gene WormBase Gene ID(s) [e.g. WBGene00000001] celegans_gene_ensembl ENSEMBL_MART_ENSEMBL
#> 9 wormbase_gseqname WormBase Gene Sequence-name ID(s) [e.g. WBGene00000001] celegans_gene_ensembl ENSEMBL_MART_ENSEMBL
#> 10 wormbase_locus WormBase Locus ID(s) [e.g. WBGene00000001] celegans_gene_ensembl ENSEMBL_MART_ENSEMBL
#> # ℹ 62 more rows
After scrolling through the results, we observe that the attribute
wormbase_cds
should work as the IDs are formatted in the
2L52.1a.1
-form.
Next, we convert the GeneID labels from uniprot to wormbase using
"wormbase_cds"
as the attribute.
# here we use the dataset phylomapr::Caenorhabditis_elegans.PhyloMap
Celegans.PhyloMap <- phylomapr::Caenorhabditis_elegans.PhyloMap
Celegans.PhyloMap.wormbase <-
phylomapr::convertID(
phylomap = Celegans.PhyloMap,
mart = "ENSEMBL_MART_ENSEMBL",
dataset = "celegans_gene_ensembl",
attributes = c("wormbase_cds"),
filters = "uniprot_gn_id")
#> Starting Gene ID conversion...
#> Starting BioMart query ...
#>
#>
#> Please cite: Drost HG, Paszkowski J. Biomartr: genomic data retrieval with R. Bioinformatics (2017) 33(8): 1216-1217. doi:10.1093/bioinformatics/btw821.
> Celegans.PhyloMap
# A tibble: 19,818 × 2
Phylostratum GeneID <dbl> <chr>
1 2 sp|A0A061ACU2|PIEZ1_CAEEL
2 3 sp|A0A078BQN7|FKH3_CAEEL
3 1 sp|A0A078BQP2|GCY25_CAEEL
4 10 sp|A0A0K3AUE4|SEA2_CAEEL
5 1 sp|A0A0K3AUJ9|PRDX_CAEEL
6 1 sp|A0A0K3AV08|MLK1_CAEEL
7 1 sp|A0A0K3AWM6|MOM5_CAEEL
8 3 sp|A0A0K3AXH1|ARID1_CAEEL
9 1 sp|A0A131MBU3|IRG7_CAEEL
10 1 sp|A0A131MCZ8|CNNM3_CAEEL
# ℹ 19,808 more rows
# ℹ Use `print(n = ...)` to see more rows
> Celegans.PhyloMap.wormbase
# A tibble: 16,319 × 2
Phylostratum GeneID <dbl> <chr>
1 2 2RSSE.1a
2 2 2RSSE.1b
3 2 2RSSE.1c
4 14 3R5.1a
5 14 3R5.1b
6 1 4R79.1a
7 1 4R79.1b
8 1 AC3.10
9 11 AC3.1a
10 11 AC3.1b
# ℹ 16,309 more rows
# ℹ Use `print(n = ...)` to see more rows
Tada! While the conversion issue between databases are mitigated,
there are still some aspects to be improved. For example, we can see a
drop in the number of genes in the phylomap. Furthermore, a bit of
fidgeting with biomartr
is needed. However, this should
still be faster than running gene age inference from scratch!
Solution 2
If the helper function convertID()
in
phylomapr
doesn’t work out well, there are other solutions
involving fast and sensitive sequence alignment, though the sequences
must be downloaded. To achieve this,
diamond_protein_to_protein_best_hits()
or
diamond_protein_to_protein_best_reciprocal_hits()
in
rdiamond
(or diamond_best()
or
diamond_rec()
in orthologr
) can be used. Here,
we show the example with
rdiamond::diamond_protein_to_protein_best_hits()
.
Setting up the example
To set up the example, we download the fasta sequences of each
proteome using biomartr
.
file_path.ENSEMBL <- biomartr::getProteome(
db = "ensembl",
organism = "Caenorhabditis elegans",
path = file.path("_ensembl_downloads","proteomes") )
file_path.UNIPROT <- biomartr::getProteome(
db = "uniprot",
organism = "Caenorhabditis elegans",
path = file.path("_uniprot_downloads","proteomes") )
Running solution for C. elegans
Now run we run
rdiamond::diamond_protein_to_protein_best_hits()
to obtain
the best hits
# obtain the best hit from ENSEMBL in the UNIPROT dataset
best_rec_hits <- rdiamond::diamond_protein_to_protein_best_hits(
query = file_path.ENSEMBL,
subject = file_path.UNIPROT,
sensitivity_mode = "fast", # change to ultra-sensitive to increase time.
cores = 8)
# select only subject and query
ENSEMBL_to_UNIPROT <- best_rec_hits %>%
dplyr::filter(perc_identity == 100) %>% # filter hits with percent identity 100% (assuming no misalignments have occured)
dplyr::select(1,2) %>%
dplyr::rename(ensembl = 1) %>%
dplyr::rename(GeneID = 2)
# replace previous UNIPROT IDs with the new ENSEMBL IDs
Celegans.PhyloMap.UNIPROT <- phylomapr::Caenorhabditis_elegans.PhyloMap
Celegans.PhyloMap.ENSEMBL <- Celegans.PhyloMap.UNIPROT %>%
dplyr::left_join(ENSEMBL_to_UNIPROT) %>% # join the ENSEMBL IDs to UNIPROT PhyloMap
tidyr::drop_na() %>% # remove rows that do not have corresponding IDs
dplyr::select(-GeneID) %>% # remove the previous UNIPROT IDs
dplyr::rename(GeneID = ensembl) # rename the ensembl column as the GeneID
Tada! Previously, the phylomap had UNIPROT IDs, i.e.
> Celegans.PhyloMap.UNIPROT
# A tibble: 19,818 × 2
Phylostratum GeneID <dbl> <chr>
1 2 sp|A0A061ACU2|PIEZ1_CAEEL
2 3 sp|A0A078BQN7|FKH3_CAEEL
3 1 sp|A0A078BQP2|GCY25_CAEEL
4 10 sp|A0A0K3AUE4|SEA2_CAEEL
5 1 sp|A0A0K3AUJ9|PRDX_CAEEL
6 1 sp|A0A0K3AV08|MLK1_CAEEL
7 1 sp|A0A0K3AWM6|MOM5_CAEEL
8 3 sp|A0A0K3AXH1|ARID1_CAEEL
9 1 sp|A0A131MBU3|IRG7_CAEEL
10 1 sp|A0A131MCZ8|CNNM3_CAEEL
# ℹ 19,808 more rows
# ℹ Use `print(n = ...)` to see more rows
Now, we have ENSEMBL gene IDs.
> Celegans.PhyloMap.ENSEMBL
# A tibble: 26,425 × 2
Phylostratum GeneID <dbl> <chr>
1 2 C10C5.1g.1
2 2 C10C5.1i.1
3 2 C10C5.1k.1
4 2 C10C5.1l.1
5 1 Y105C5B.2a.1
6 10 K10G6.3a.1
7 10 K10G6.3c.1
8 10 K10G6.3e.1
9 10 K10G6.3f.1
10 10 K10G6.3g.1
# ℹ 26,415 more rows
# ℹ Use `print(n = ...)` to see more rows
The main issue with solution 2 is the increased number of
dependencies, including the sequence aligner DIAMOND
,
though one can imagine that other aligners could be used such as
MMSeqs2
or BLAST
. The most important thing
here is that the corresponding IDs can be obtained and that users cite
the package/software that has been used.